Business Analytics: Everything You Need to know
- Pankaj kumawat
- May 3, 2020
- 13 min read
Business analytics is everywhere. Learn about what business analytics is, components, types of BA, challenges, careers, popular tools, and latest trends.
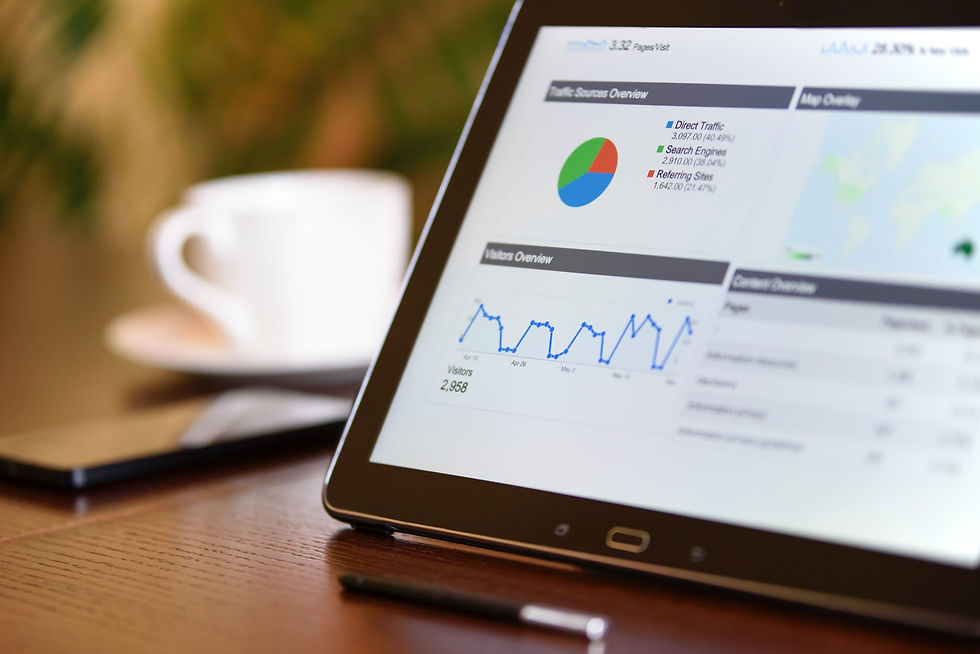
Definition: What is Business Analytics?
Business analytics is the process of collating, sorting, processing, and studying business data, and using statistical models and iterative methodologies to transform data into business insights. The goal of business analytics is to determine which datasets are useful and how they can be leveraged to solve problems and increase efficiency, productivity, and revenue.
A subset of business intelligence (BI), business analytics is generally implemented with the goal of identifying actionable data. Business intelligence is typically descriptive, focusing on the strategies and tools utilized to acquire, identify, and categorize raw data and report on past or current events. Business analytics is more prescriptive, devoted to the methodology by which the data can be analyzed, patterns recognized, and models developed to clarify past events, create predictions for future events, and recommend actions to maximize ideal outcomes.
Sophisticated data, quantitative analysis, and mathematical models are all employed by business analysts to engineer solutions for data-driven issues. They can utilize statistics, information systems, computer science, and operations research to expand their understanding of complex data sets, and artificial intelligence, deep learning, and neural networks to micro-segment available data and identify patterns. This information can then be leveraged to accurately predict future events related to consumer action or market trends and to recommend steps that can drive consumers toward a desired goal.
Components of Business Analytics
Mobile dashboards have similar components to business dashboards, but with a few key differences. The components of business dashboards include:

Data Aggregation
Data Mining
Association and Sequence Identification
Text Mining
Forecasting
Predictive Analytics
Optimization
Data Visualization
Data Aggregation Before data can be analyzed, it must be collected, centralized, and cleaned to avoid duplication, and filtered to remove inaccurate, incomplete, and unusable data. Data can be aggregated from:
Transactional records: Records that are part of a large dataset shared by an organization or by an authorized third party (banking records, sales records, and shipping records).
Volunteered data: Data supplied via a paper or digital form that is shared by the consumer directly or by an authorized third party (usually personal information).
Data Mining In the search to reveal and identify previously unrecognized trends and patterns, models can be created by mining through vast amounts of data.
Data mining employs several statistical techniques to achieve clarification, including:
Classification: Used when variables such as demographics are known and can be used to sort and group data
Regression: A function used to predict continuous numeric values, based on extrapolating historical patterns
Clustering: Used when factors used to classify data are unavailable, meaning patterns must be identified to determine what variables exist
Association and Sequence Identification In many cases, consumers perform similar actions at the same time or perform predictable actions sequentially. This data can reveal patterns such as:
Association: For example, two different items frequently being purchased in the same transaction, such as multiple books in a series or a toothbrush and toothpaste.
Sequencing: For example, a consumer requesting a credit report followed by asking for a loan or booking an airline ticket, followed by booking a hotel room or reserving a car.
Text Mining Companies can also collect textual information from social media sites, blog comments, and call center scripts to extract meaningful relationship indicators. This data can be used to:
Develop in-demand new products
Improve customer service and experience
Review competitor performance
Forecasting A forecast of future events or behaviors based on historical data can be created by analyzing processes that occur during a specific period or season. For example:
Energy demands for a city with a static population in any given month or quarter
Retail sales for holiday merchandise, including biggest sales days for both physical and digital stores
Spikes in internet searches related to a specific recurring event, such as the Super Bowl or the Olympics
Predictive Analytics Companies can create, deploy, and manage predictive scoring models, proactively addressing events such as:
Customer churn with specificity narrowed down to customer age bracket, income level, lifetime of existing account, and availability of promotions
Equipment failure, especially in anticipated times of heavy use or if subject to extraordinary temperature/humidity-related stressors
Market trends including those taking place entirely online, as well as patterns which may be seasonal or event-related
Optimization Companies can identify best-case scenarios and next best actions by developing and engaging simulation techniques, including:
Peak sales pricing and using demand spikes to scale production and maintain a steady revenue flow
Inventory stocking and shipping options that optimize delivery schedules and customer satisfaction without sacrificing warehouse space
Prime opportunity windows for sales, promotions, new products, and spin-offs to maximize profits and pave the way for future opportunities
Data Visualization Information and insights drawn from data can be presented with highly interactive graphics to show:
Exploratory data analysis
Modeling output
Statistical predictions
These data visualization components allow organizations to leverage their data to inform and drive new goals for the business, increase revenues, and improve consumer relations.
Types of Business Analytics
There are four types of business analytics, each increasingly complex and closer to achieving real-time and future situation insight application. These analytics types are usually implemented in stages, starting with the simplest, though one type is not more important than another as all are interrelated.
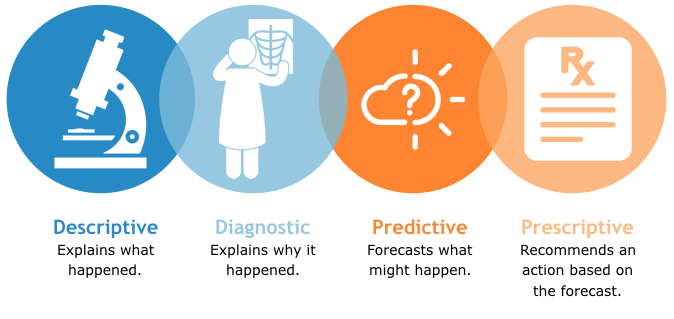
The following BA examples provide insight into the roles of each type in the analytics process. By leveraging these four types of analytics, big data can be dissected, absorbed, and used to create solutions for many of the biggest challenges facing businesses today.
Descriptive Analytics Descriptive analytics describes or summarizes a business’s existing data to get a picture of what has happened in the past or is happening currently. It is the simplest form of analytics and employs data aggregation and mining techniques. This type of business analytics applies descriptive statistics to existing data to make it more accessible to members of an organization, from investors and shareholders to marketing executives and sales managers. Descriptive analytics can help identify strengths and weaknesses and provide insight into customer behavior. Strategies can then be developed and deployed in the areas of targeted marketing and service improvement, albeit at a more basic level than if more complex diagnostic procedures were used. The most common physical product of descriptive analysis is a report heavy with visual statistical aids.
Diagnostic Analytics Diagnostic analytics shifts from the “what” of past and current events to “how” and “why,” focusing on past performance to determine which factors influence trends. This type of business analytics employs techniques such as drill-down, data discovery, data mining, and correlations to uncover the root causes of events. Diagnostic analytics uses probabilities, likelihoods, and the distribution of outcomes to understand why events may occur and employs techniques including attribute importance, sensitivity analysis, and training algorithms for classification and regression. However, diagnostic analysis has limited ability to provide actionable insights, delivering correlation results as opposed to confirmed causation. The most common physical product of diagnostic analysis is a business dashboard.
Predictive Analytics Predictive analytics forecasts the possibility of future events using statistical models and machine learning techniques. This type of business analytics builds on descriptive analytics results to devise models that can extrapolate the likelihood of select outcomes. Machine learning experts and trained data scientists are typically employed to run predictive analysis using learning algorithms and statistical models, enabling a higher level of predictive accuracy than is achievable by business intelligence alone. A common application of predictive analytics is sentiment analysis. Existing text data can be collected from social media to provide a comprehensive picture of opinions held by a user. This data can be analyzed to predict their sentiment towards a new subject (positive, negative, neutral). The most common physical product of predictive analysis is a detailed report used to support complex forecasts in sales and marketing.
Prescriptive Analytics Prescriptive analytics goes a step beyond predictive analytics, providing recommendations for next best actions and allowing potential manipulation of events to drive better outcomes. This type of business analytics is capable of not only suggesting all favorable outcomes according to a specified course of action, but recommending specific actions to deliver the most desired result. Prescriptive analytics relies on a strong feedback system and constant iterative analysis and testing to continually learn more about the relationships between different actions and outcomes. One of the most common uses of prescriptive analytics is the creation of recommendation engines, which strive to match options to a consumer’s real-time needs. The key to effective prescriptive analysis is the emergence of deep learning and complex neural networks, which can micro-segment data across multiple parameters and timelines simultaneously. The most common physical product of prescriptive analysis is a focused recommendation for next best actions, which can be applied to clearly identified business goals. These four different types of analytics may be implemented sequentially, but there is no mandate. In many scenarios, organizations may jump directly from descriptive to prescriptive analytics thanks to artificial intelligence, which streamlines the process.
Business Analytics Examples
When it comes to business analytics, success often depends on whether or not all parties of an organization fully support adoption and execution. Successful BA examples—and subsequent deployment of new predictive-based initiatives—include:
Predictive Maintenance: Shell
Royal Dutch Shell PLC recently implemented predictive maintenance driven by artificial intelligence to cut down on time lost to machine failure. The AI-powered tools predict when maintenance is needed on compressors, valves, and other equipment, can autonomously analyze data to help steer drill bits through shale deposits, and will soon be able to identify and alert station employees of dangerous behavior by customers, reducing risks from the drilling platform to the gas pump. The systems can anticipate when and where more than 3,000 different oil drilling machine parts might fail, keep Shell informed about the location of parts at their worldwide facilities, and plan when to make purchases of machine parts. These systems also determine where to place inventory items and how long to keep parts before putting them into rotation or replacing/returning them. Shell has since reduced inventory analysis from over 48 hours to less than 45 minutes, saving millions of dollars each year thanks to reduced costs of moving and reallocating inventory.
Predictive Deliveries: Pitt Ohio
Pitt Ohio, a $700 million freight company, was significantly impacted by Amazon’s same-day delivery initiative, which ramped up customer expectations. Customers also became more demanding, requesting up-to-the-minute tracking and estimated times of delivery that were much narrower than formerly acceptable windows. The company turned to data analysis to find a way to improve customer experiences. A cross-departmental project involving market research, sales operations, and IT was launched internally, leveraging data that was previously unused. The historical data, predictive analytics, and algorithms that calculated freight weight, driving distance, and several other factors in real-time allowed Pitt Ohio to estimate delivery times at a 99 percent accuracy rate. The company estimates that repeat orders increased its revenue by $50,000 per year, and customer churn reduction equaled retained revenues of $60,000 per year.
Predictive Banking: Axis Bank
Axis Bank, the third-largest private sector bank in India, implemented robotics process automation and deep learning to identify customer behavioral patterns and recommend next best actions to prevent customer churn, including streamlining document processing, identifying “events” when customers were more likely to leave, and preemptively offering special promotions targeted to those segmented audiences to prevent churn. For better customer experience, 125 “customer journeys” were identified, analyzed, and retooled, and time spent verifying customer-provided data across multiple documents in the back office dropped from 15 minutes to 2–3 minutes. Axis is now developing a chatbot to speed customer interactions and reduce wait times for service at busy branches and during peak interface times.
Challenges Presented by Business Analytics
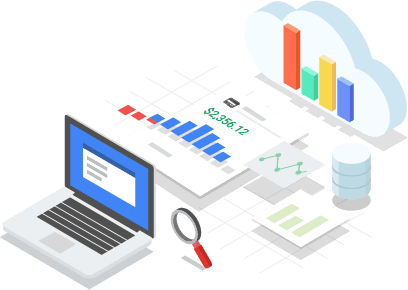
When it comes to business analytics, success often depends on whether or not all parties of an organization fully support adoption and execution. Successful BA examples—and subsequent deployment of new predictive-based initiatives—include:
Executive Distrust Getting everyone in upper management to sign off on BA implementation can be difficult. Although most organizations have embraced some form of BI and are likely handling data warehousing effectively, analytics is still an area viewed with distrust by many top-level executives, and trust must be built to effectively leverage data analysis. Presenting business analytics as supportive to existing company strategies and outlining clear, measurable goals can help convince slow adopters to approve a trial project.
Poor Collaboration Failure to achieve teamwork among a cross-section of departments can cripple the evaluation and implementation of analytics-driven initiatives. Business and IT personnel must be in-sync for an analytics strategy to succeed. Poor collaboration creates the risk that analytics won’t provide the information promised, leading to further distrust and potential abandonment of beneficial technology innovation. A cross-functional analytics team that includes major players in technology, business, operations, legal, and HR can help full-scale adoption of analytics in every department.
Lack of Commitment While many analytics software packages are presented as a prefabricated solution that is easy to implement, cost can be discouraging and return on investments are often not immediate. Although analytical models develop over time and predictions will improve, dedication is required during the initial months of an analytics initiative. Businesses that fail to make it through this crucial investment period may see executives losing trust in the solution and refusing to believe the models, eventually abandoning the concept. Process and goal owners must establish a productive analytics environment and set realistic timelines for results.
Slow Information Maturity BA implementations often fail due to lack or low quality of available data. A maturity assessment should always be performed on the company’s information architecture and data sources based on analytical requirements. Transactional, aggregated, and operational information should be scored for quality, and the existing integration infrastructure’s ability to support new sources and data feed should be evaluated. The time required to acquire, clean, and analyze new data must be built into the adjustment period.
Business Analytics Tools
BA tools include many methodologies and open source solutions that can be leveraged to help analysts perform tasks and generate reports that are easy for laypersons to understand.
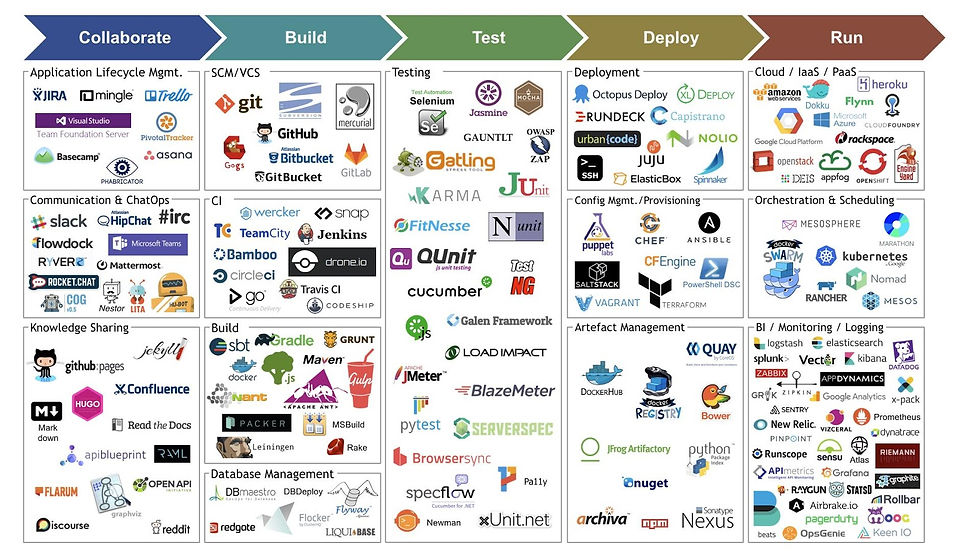
Requirement management (RM) tools help ensure that organizations can identify, document, verify, and meet the needs and expectations of their target demographics and existing customers. Requirements may be generated from customers, partners, or stakeholders. Many companies simply use Microsoft Excel at a business-wide level for RM.
Reporting tools can be obtained from open source platforms to allow business analysts to manage their processes and present their findings in a relevant, comprehend-able way. Some of the most flexible and user-friendly open source options include:
Tableau offers intelligent information representation items dependent on business insight. The product offers shading, movement, and cartography ability to make information perceptions as simple as would be prudent.
Tableau interfaces an extensive variety of information sources, from setting up sources like Excel to ongoing augmentations like Amazon Aurora, Microsoft Azure SQL Data Warehouse and MapR Hadoop Hive Connectivity for Mac.
Tableau rushes to actualize and its representation stretches out into the overseer devices, for example, visual permissioning and administration.
Power BI is an arrangement of business expository tools to convey bits of knowledge to the association. It gives intelligent perceptions and self-benefit BI capacities where end clients can make reports and dashboards without anyone else’s input.
Power BI conveys information stockroom abilities including information planning, information disclosure, and an intuitive dashboard. Power BI likewise has an extra administration installed on its Azure cloud stage.
Likewise, it can stack custom perceptions as per the business necessities.
Positioned among the best Business Intelligence tools in the BI advertise, Sisense is generally known for its ability to amazingly compose information from numerous sources without expensive arrangements.
Sisense, one of the Business Intelligence arrangements, works with the imaginative ElastiCube innovation, to import huge arrangements of information and work with any CPU design without trading off the nature of your outcomes.
Careers in Business Analytics

A career in business analytics usually requires at least a bachelor’s degree in business analytics, data science, information management, applied analytics, business intelligence, statistics, marketing, or a related field. A master’s degree can widen job choices and certifications can provide more authority and help increase value. BA experts often report directly to upper management within their organization. They may be in a department of their own but coordinate closely with sales, marketing, and operations. Careers available in business analytics include (but are not limited to):
Management Analyst/Consultant
Management analysts and consultants work to ensure that their organization’s business operations are running efficiently. This job typically requires working closely with all departments to identify which business processes can be improved, find solutions to enhance efficiency, and communicate recommendations to upper management.
Data Analyst/Scientist
Data analysts and scientists are expected to collect, organize, and analyze data to determine how to leverage insights for their organization. This includes using mathematical models and other tools to determine what data is useful and deliver actionable recommendations. In most cases, a full report complete with visual tables, charts, and reports must be presented to upper management to inform their decision-making processes.
Business Intelligence Analyst
BI analysts are tasked with gathering and analyzing information to gain an advantage in their organization’s associated market. The analysis should show upper management a clear picture of where the business stands and its strengths and weaknesses, guiding decisions for higher profitability.
Program and Marketing Managers
Programming and marketing managers generally have the responsibility of developing and deploying their organization’s marketing strategies. In many cases, this means managing their organization’s entire sales and marketing staff, overseeing all marketing initiatives and campaigns, and reporting to and advising upper management.
Big Data Analytics Specialist
Big data analytics specialists utilize the latest in modern technology and data science to solve the challenges presented by an increasingly digital landscape. This position will be expected to deliver improved business performance and to weigh in on data-driven decision-making within their organization.
Operations Research Analyst
Operations research analysts are responsible for analyzing their organization’s operational data. This typically requires using information technology to complete analysis and develop solutions for improving process efficiency across multiple departments. This position may also include the responsibility of creating and presenting a comprehensive report to stakeholders.
Market Research Analyst
Market research analysts are expected to collate, manage, and derive insights from vast amounts of marketing data for their organization. This data analysis should help identify potential customers and price points and evaluate product desirability, enabling the company to increase revenues consistently over time.
Business Analytics Certifications
Business Analytics Course Online by Babson College is an introductory certification developed to teach the hands-on skills and knowledge required to analyze data, reach meaningful conclusions, and present findings to business executives. It covers data aggregation, visualization, descriptive statistics, basic probability, statistical inference, and linear models.
The Statistics for Business Analytics A–Z course is designed for recent graduates seeking to build a career as a data scientist or business analyst and provides practical approaches to solving business problems with large datasets by delivering a grasp of distributions, central limit theorem, and statistical significance.
The Business Analytics Certification is a MicroMasters program that delivers the skills and understanding of analytics required to improve business performance using data, quantitative and statistical analysis, and predictive modeling. Computing tools, mathematical models, and statistical analysis are employed to predict business decision outcomes and identify next best actions.
The Wharton Business Analytics Certificate introduces big data analytics for professionals of all experience levels, introducing the processes used to describe, predict, and inform strategic, data-driven business decisions across marketing, human resources, operation, and finance. Wharton’s Coursera also offers an Advanced Business Analytics Course for analysts seeking an advanced specialization to utilize existing data analytics skills to increase profits by extracting and manipulating data, executing statistical methods for descriptive, diagnostic, predictive, and prescriptive analysis, and presenting findings in an executive/shareholder-friendly format. The Strategic Business Analytics program is designed for students, analysts, and data scientists seeking to apply their statistical knowledge to business contexts. A background in statistics or another programming language and familiarity with data analysis is a prerequisite for this advanced training class, which focuses on developing BI insights and applying them to enterprise-scale problems.
Trends in Business Analytics
Big Data With an increasing emphasis on digitization in every aspect of life, datasets continue to expand at an unprecedented rate. This expansion is both an advantage and a disadvantage—more data means more potential insights, but the sheer volume can be overwhelming.
Artificial Intelligence As AIs become smarter and able to teach themselves, AIs created by AIs are being developed and launched in industry verticals such as banking, financial services, insurance, retail, hospitality, engineering, manufacturing, and more.
Deep Learning The next step up from machine learning, deep learning leverages the advantages of vast computing power to manage enormous data sets, identifying patterns and delivering predictive results that were formerly impossible.
Neural Networks Data scientists can now create “brains” which have the computing power of thousands of human minds. Data can be processed and sorted, patterns can be identified along a historical timeline, and future predictions are delivered with an unprecedented level of accuracy.
The Internet of Things IoT-driven devices number in the millions, delivering real-time data to organizations worldwide and allowing intimate entry into the lives of consumers around the globe.
Micro-Segmentation As data becomes bigger, the ability to separate it into smaller and smaller slices enables organizations to accurately define their “ideal” customer and create funnels that lead them directly to the desired action. This granular segmentation is the driving force behind successful digital transformation initiatives.
Comments